Should I trust my data?
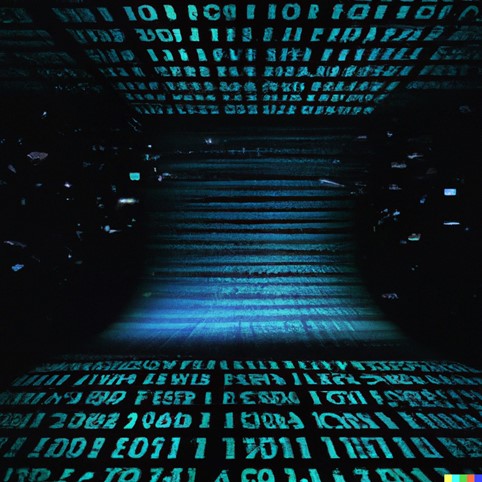
"Data is like garbage. You'd better know what you are going to do with it before you collect it." - Mark Twain
There is hype going on around data, data science, and the outputs of machine learning. Every company gathers data without being sure if they will use it, just in case. Then we obtain a new cool term which is Dark Data. It refers to the data collected, processed, and stored but not used for any meaningful purposes. It is estimated that at least 2.5 quintillion bytes (or 2.5 billion gigabytes) of data is produced daily, and more than half of all data is dark.
Let us assume we want to break this chain and use the gathered data. How can we trust it? There are several factors that can affect the trustworthiness of data. The high-quality data can possess the following properties:
- Accuracy: The data should be correct and free from errors. The accuracy of data can be measured by comparing it with a known standard or by using statistical methods to assess the likelihood of errors.
- Completeness: The data should be comprehensive and include all relevant information. Incomplete data can result in inaccurate analysis and decision-making.
- Consistency: The data should be consistent across different sources, time periods, and locations. Inconsistencies can lead to confusion and errors in analysis.
- Relevance: The data should be relevant to the intended use and purpose. Irrelevant data can distract from important information and reduce the effectiveness of analysis.
- Timeliness: The data should be up-to-date and available when needed. Outdated or delayed data can result in missed opportunities or incorrect decisions.
- Validity: The data should be based on sound methods of collection and processing. Validity can be assessed by examining the methodology used to collect and process the data.
- Accessibility: The data should be easy to access and use. This can include factors such as the format of the data, the ease of accessing it, and the level of technical expertise required to work with the data.
These are all excellent features, but most of the time (all the time), we cannot have it all and need to clean the data. If you have relatively low-volume data, you can observe the outliers with visualization techniques. Unfortunately, problems with the data may not be visible to the human eye. In these circumstances, you need to question everything you know about the data I like Sheldon's following scene in The Big Bang Theory tv series. I think it summarizes the data analysis process very successfully.
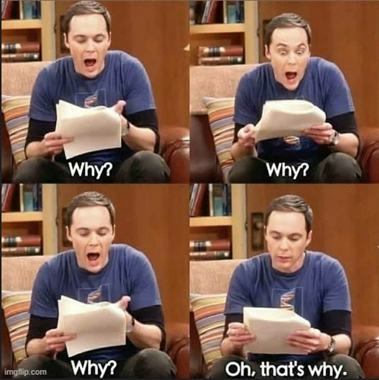
While examining of trustworthiness of data, discussing data with the domain expert is always helpful because most of the time, while examining the data, we only see rows and columns, but they are the result of an action. Domain experts can identify irrelevant data and provide a context around the data, including the history and background of the industry, key trends, and any external factors that may be influencing the data. Also, they can help identify patterns in the data and any outliers or anomalies that may be important to investigate further. For this reason, I firmly believe in the collaboration between domain experts.
In summary, it is obvious that while data can be a valuable tool in making informed decisions, it is essential to approach it with a critical eye, recognizing that data may be subject to limitations and biases. Furthermore, data should be used in conjunction with other sources of information to gain a more comprehensive understanding of the issue at hand. By combining data with expert opinions, industry knowledge, market trends, and other relevant information, decision-makers can develop a more complete picture of the situation and make informed decisions that are grounded in a well-rounded understanding of the issue.