Uncovering the Hidden Biases: Diving Into The Complex Relationship Between Bias and AI
Associated with the overwhelming digital transformation of most industries, artificial intelligence (AI) is transforming our world in numerous ways, from healthcare and transportation to entertainment and education. However, the sense of losing the Human common sense to the automation of the machine brings up one issue that has arisen with the increasing use of AI: the problem of bias. It can be present in the historical data that we use to learn our algorithms, or in the way we collect it and process, or even in the configuration of the AI algorithm itself. Bias in AI refers to the tendency of AI systems to produce results that reflect the prejudices and assumptions of their human creators. In this discussion forum post, we will explore the complex relationship between bias and AI.
There have been plenty of examples on how biased data affects automation bias, e.g., transferring to Amazon’s automated recruitment system (later dismissed in 2017) the underrepresentation of Women in STEM (science, technology, engineering and mathematics); to US healthcare algorithm (serving over 200 million people) the underestimated medical needs of black patients; or Microsoft’s 2016 attempt to showcase an AI chatbot assimilating the internet's worst tendencies into its personality. These examples are now old and have multiplied across not so well thought out implementations of AI-based methodologies that have impact on our lives. It is also true that we are evermore knowledgeable in building AI systems and also in what to take into account when doing that.
The first step in understanding bias in AI is to recognize that all AI systems are created by humans, who are themselves subject to biases and prejudices, sometimes hidden and often unintentional. As a result, it is difficult to completely eliminate bias from AI systems. However, there are steps that can be taken to minimize bias and ensure that AI systems are as fair and impartial as possible. One way to minimize bias in AI is to use diverse datasets that reflect a range of experiences and perspectives. If an AI system is trained on a narrow dataset that only represents a certain segment of the population, it is more likely to produce biased results. For example, if an AI system is trained on data that is predominantly male, it may be more likely to make biased decisions when it comes to issues that affect women.
Another way to minimize bias in AI is to use transparency and accountability mechanisms. If AI systems are designed to be transparent, it is easier to identify and correct biases as they arise. Additionally, accountability mechanisms can help to ensure that AI systems are used responsibly and ethically. For example, if an AI system is used to make decisions about employment or lending, there should be oversight mechanisms in place to ensure that the system is not making biased decisions. However, it is important to recognize that bias in AI is not always intentional. In some cases, AI systems may produce biased results simply because the data they are trained on contains biases. For example, if an AI system is used to predict the likelihood of someone being a criminal, and the data it is trained on is biased against certain racial or ethnic groups, the system may produce biased results even if there was no intention to do so.
To address this issue, it is important to recognize the limitations of AI and to use it in conjunction with other tools and approaches. AI should be seen as a complement to human decision-making, rather than a replacement for it. Human oversight and intervention are necessary to ensure that AI systems are being used in a fair and ethical manner. With the International Research Centre on AI under the auspices of UNESCO, IRCAI, we are working with the European Commission on a project - AI4Gov - engaging eleven other institutions from seven countries to explore the potential of AI and Big Data technologies for developing evidence-based innovations, policies, and policy recommendations It contributes harness the public sphere, political power, and economic power for democratic purposes. The project intends to contribute to the research landscape that addresses ethical, trust, discrimination, and bias issues, and provide solutions to the challenges faced by stakeholders in modern democracies. The project will utilize the public sphere, political power, and economic power to promote democratic goals. It seeks to enhance the research landscape by addressing ethical concerns, trust issues, discrimination, and bias, and providing solutions to the challenges encountered by stakeholders in contemporary democracies. The first workshop of this project happens this week in May 16 from 12:00 CEST, over an hybrid event, accessible online here, joining the efforts of research institutions, the ministry and NGOs.
The engagement of policy makers on these matters is fundamental, not only to leverage their extended experience and better understand how to deal with the practical aspects of avoiding bias and unfairness, but also to engage them in eliminating that bias from the data sampling and algorithm design. A while ago we took the role of AI partner in the European Commission project MIDAS aspiring to create, develop, and provide functional Big Data technologies empowering policymakers to make better-informed decisions by utilizing actionable insights from a diverse range of healthcare and related data. This challenge entails multi-disciplinary research, encompassing policy development, technology, and advancements in deploying data effectively to assist in policy revision. In this project we have analysed together with policy-makers and health professionals from several European countries the specific challenges in the health sector, expressed in the worldwide news often due to the complexity of the scientific information the topics are based on, even without any intentional manipulation or ideology impact. As discussed in my paper on Bias in Health reflecting some of these observations, inaccuracy and misunderstanding can be present in the chain of interpretations of the data and the results of the algorithms. By analysing the healthcare news we can see the different impact, e.g., in the coverage of Ebola in African countries compared with the first (and only) death case identified in the USA in 2015. The initiative of Google to track the evolution of Influenza based on search queries, Google Flu Trends, showed also divergent results when the news start alarming the population on the intensity of the epidemics. The bias has always been much present in the public opinion discussion about vaccines, which was a main controversy in the times of COVID-19.
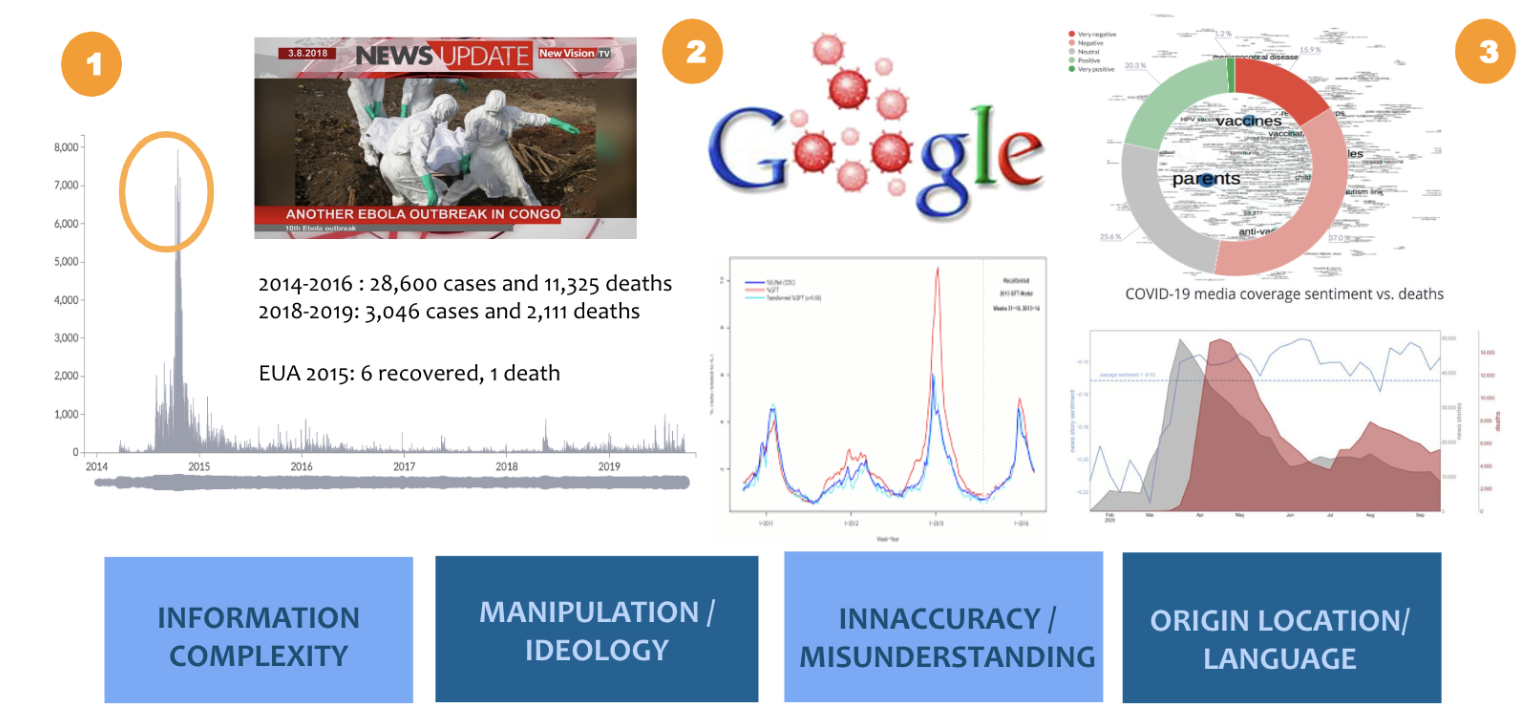
Bias in AI is a complex issue that requires careful consideration and attention. While it is almost mission impossible to completely eliminate bias from AI systems, there are steps that can be taken to minimize it and ensure that AI systems are as fair and impartial as possible. By recognizing the limitations of AI and using it in conjunction with other tools and approaches, we can harness the power of AI while minimizing its potential for harm. Share with us your experiences and your opinion about this topic here in the discussion forum!