Riding the Storm: How AI is Can Contribute to A More Efficient Response to Extreme Weather
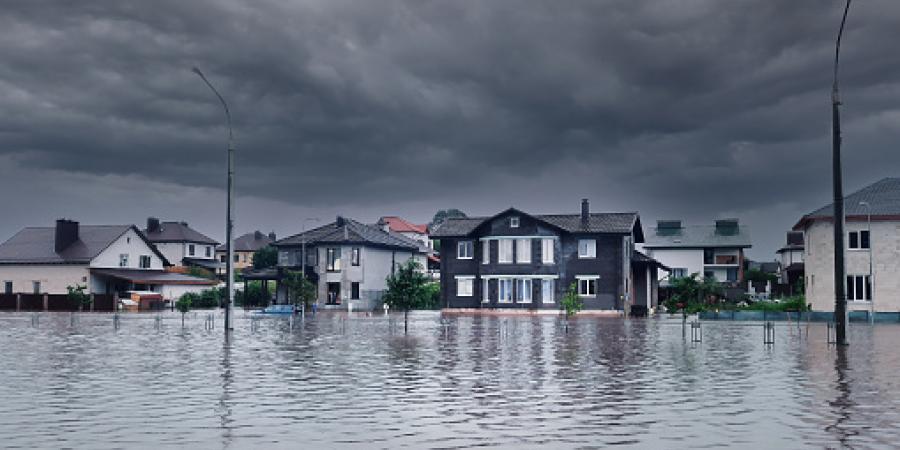
Extreme weather events such as hurricanes, floods, and wildfires are becoming more frequent and severe due to climate change. These disasters can cause extensive damage to infrastructure, loss of life, and disrupt economic and social systems. In such situations, early detection and rapid response are critical for mitigating the impact of these events. But it is also useful to use data-driven methods to have an early overall assessment of the impact caused by the natural event. Artificial intelligence (AI) has emerged as a powerful tool to help manage these disasters by providing accurate predictions, efficient communication, and effective decision-making. With the satellite imagery resources becoming more available in better frequency, and a wide range of specialized sensors getting cheaper and more customizable, there is an opportunity for data-driven technologies that employ AI to become part of the answer to the aspects of climate change preparedness relating to these extreme weather events. But in this post we will also discuss the use of text mining methods to explore the insights captured in media, social media and published science to contribute to a more complete understanding of the behavior change of climate, and the best practices that are making the change across the world.
One of the primary applications of AI in extreme weather events is in predicting the onset and severity of these events, using machine learning algorithms to analyze vast amounts of data, including weather patterns, atmospheric conditions, and historical weather data, to identify patterns and predict when and where extreme weather events are likely to occur. There are a few well established Geographic Information Systems (GIS) that allow for dynamic layers of information providing insight and forecasts on, e.g., water levels in time and other water related information. It is useful to have real time data on these matters, that can be provided by, e.g., the European Centre for Medium-Range Weather Forecasts relying on the Copernicus satellite imaging, or earth observation solutions like the Bluedot Water Observatory, but is also useful to be able to go back in time in search for trends and change of behaviors, to which the Global Surface Water Explorer, developed by European Commission’s Joint Research Center, is a great example of. This analysis, heavily assisted by AI and computer vision state-of-the-art methods, can help governments and emergency responders better prepare and respond more effectively to disasters in the context of a change of climate conditions triggering unexpected events.
During extreme weather events, communication channels often become overloaded, and it can be challenging to disseminate critical information to those affected quickly. AI-powered chatbots can help address this challenge by providing real-time updates, answering frequently asked questions, and offering guidance on how to stay safe during the disaster. This technology can also assist in coordinating rescue efforts by providing location-specific information to emergency responders. A good example of these GIS-based solutions is XLAB’s spin-off SmartLocator offering location and communication in emergency situations, already in place across several European emergency call centres. Another critical application of AI in extreme weather events is in damage assessment and recovery efforts. AI-powered systems can analyze satellite images, aerial photographs, and other data sources to identify areas that have been most affected by disasters. This information can be used to prioritize recovery efforts, allocate resources effectively, and estimate the cost of damages. This data can also help in planning for future disasters by identifying areas that are more vulnerable to extreme weather events and implementing preventative measures.
Recent advancements in machine learning have made it possible to retrieve information from textual documents in nearly real-time. This capability can assist in detecting hydrological events through news and social media, providing valuable insights into aspects such as event return period or phases of extreme weather. Additionally, this data can help enhance extreme weather event alert systems though the weaker signal of social media. This follows the contribution to the digitalisation of the water sector within the initiative NAIADES with the European Commission, earlier discussed in this post. In a recently submitted research paper (that we cannot yet disclose), we studied the relation between the impact and event characteristics that can be derived from news sources in comparison to what is later established by traditional hydrological sources. The paper explores the role of media observation worldwide in extracting and estimating hydrological characteristics of floods, droughts, and heat waves. This method complements traditional monitoring and measurement techniques as an earlier but weaker signal. Furthermore, the paper outlines the potential of text mining technologies applied to historical hydrometeorological extreme data gathered from news, the social media related to water and these kinds of extreme events through a research collaboration with Twitter, and published research articles in the Microsoft Academic Graph. This as also attracted interest in the study of landslide fenomena, motivating a collaboration with Matiaž Mikoš, the UNESCO chair for water disaster risk reduction as in the published Springer book chapter about this topic.
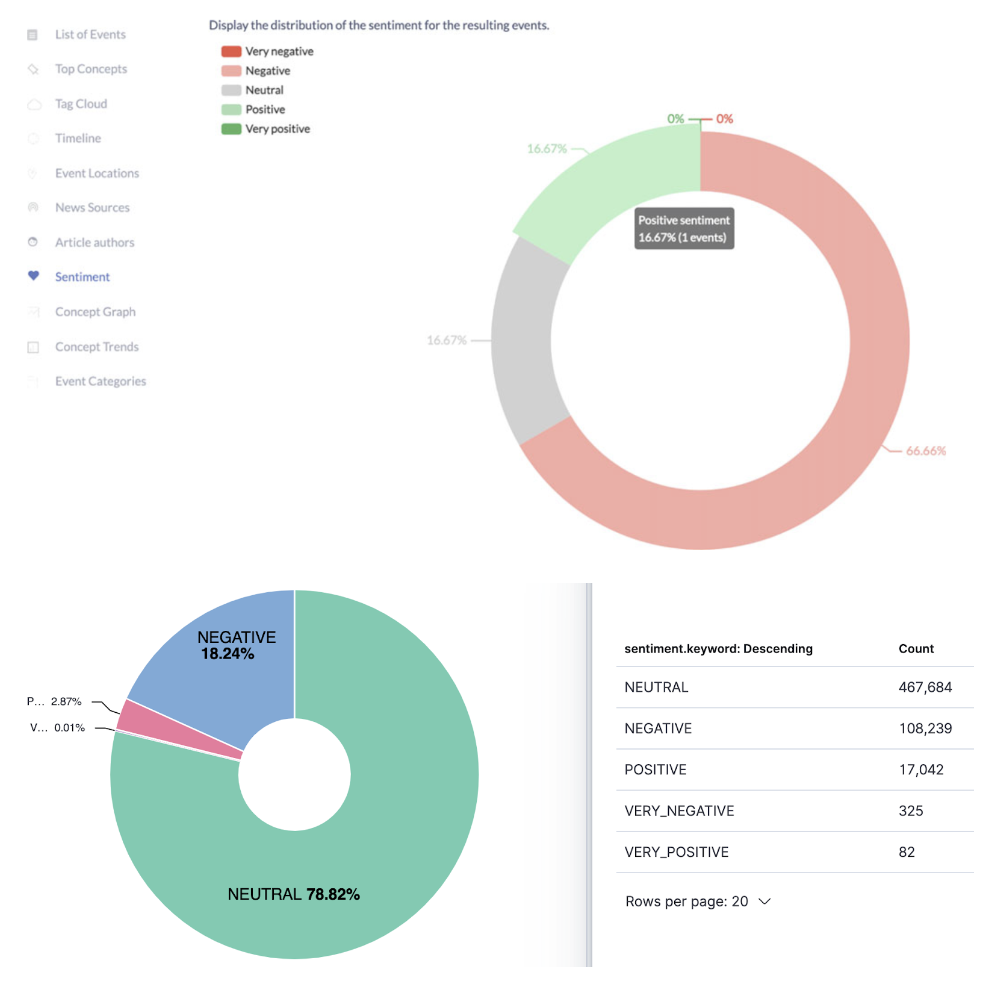
In the study we also examine how social media nowcasting can be improved by extracting hydrological event information from news data, learning new automatically identified concepts in worldwide events. Using machine learning to focus on the tweets related to the identified events and related concepts can make the detection of extreme weather events more efficient. We are also using Granger causality to relate the extracted information from social media with indicators that might help explain certain factors in the consequences of the event and help reduce its impact promoting faster and beforehand response. Furthermore, we are also calculating the sentiment in news and tweets (see figure below) to have an overall view of the perception of the different dimensions of such an extreme weather event (nobody should be too happy about a devastating flood) also capturing the public’s opinion on the effectiveness of authorities and emergency services on solving the many problems associated with the event. These applications demonstrate the enormous potential of machine learning in extracting valuable information from textual data for the purpose of improving our understanding and management of hydrological events.
By providing accurate predictions, efficient communication, and effective decision-making, AI can help governments and emergency responders prepare for and respond to disasters more effectively. While AI is not a solution for all problems, it can play a critical role in mitigating the impact of these events and improving our resilience to extreme weather events in the future. What is your opinion about this topic? Do you have further comments on the application of AI for climate change preparedness? Please share it here with us!