Unbreakable Chains: How AI is Fortifying Cyber Resilience in Supply Chains
Conventional log monitoring solutions rely on manual rule-based analysis, whereas newer AI techniques utilize Natural Language Processing to model log streams and detect normal operating patterns. However, combining the benefits of these dynamic and static monitoring methods can create a powerful automated system capable of identifying anomalous situations and potential security risks without requiring human intervention. In this article we explore the potential benefits of integrating both novel and well-established techniques, in the context of an often conservative domain.
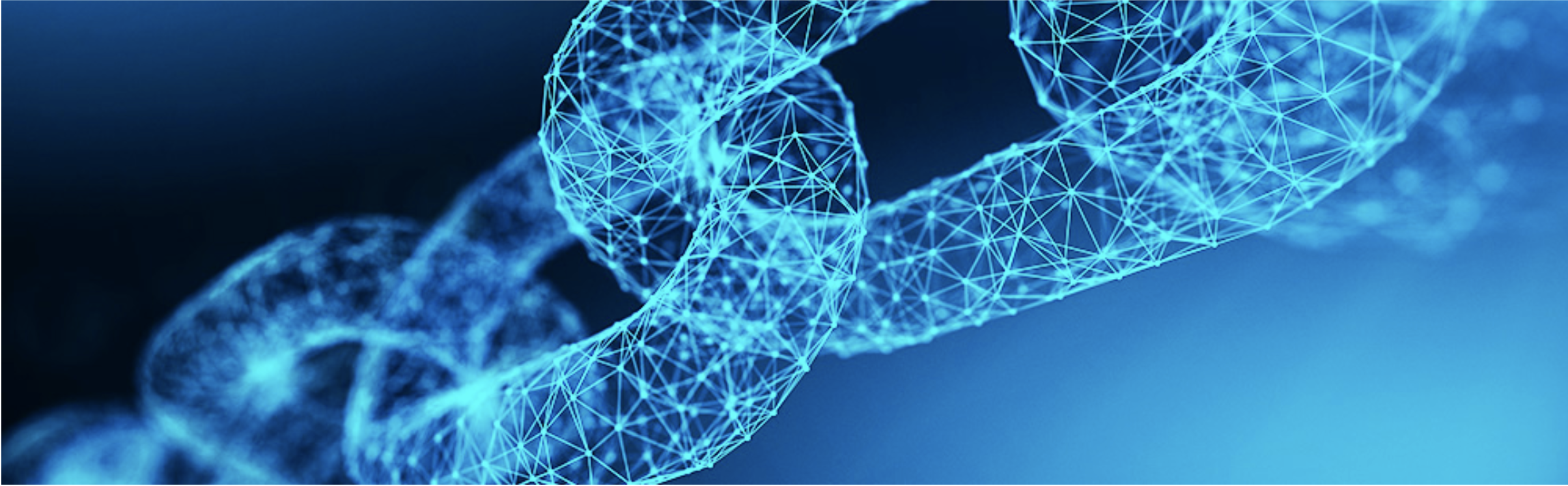
Cases like the cyberattack to the Maersk in 2017, costing up to 300 million euro, or the Solarwinds attack starting in 2019 but only disclosed in late 2020 compromising many companies, have highlighted the importance of supply chain resilience. Disruptions to global supply chains have caused significant economic and social impacts, in addition to physical disruptions, show how supply chains are vulnerable to cyber threats given their complexity and heterogeneity between stakeholders, and can have serious consequences for businesses and their customers. Artificial intelligence (AI) has the potential to play a key role in enhancing the cyber resilience of supply chains, by identifying and mitigating cyber threats in real time, allowing for a rapid response that is either triggered by the user or automatically by the system. The current state of AI for bullet-proof cyber resilience of supply chains is promising, with significant progress being made in recent years. AI-powered cybersecurity solutions are now widely available and are being used by businesses of all sizes to protect their networks and data. These solutions use advanced algorithms and machine learning techniques to detect and respond to cyber threats in real time, reducing the risk of data breaches and other cyber incidents.
One of the key benefits of AI-powered cybersecurity solutions is their ability to provide real-time threat intelligence. These solutions use advanced analytics and machine learning algorithms to analyze vast amounts of data from a range of sources, including network traffic, user behaviour, and threat intelligence feeds. This enables them to identify and respond to cyber threats in real-time, reducing the risk of data breaches and other cyber incidents. Another benefit of AI-powered cybersecurity solutions is their ability to automate many cybersecurity tasks, such as threat detection, response, and remediation. This enables businesses to respond to cyber threats more quickly and efficiently, reducing the impact of cyber incidents on their operations and customers. It also frees up cybersecurity professionals to focus on more strategic tasks, such as developing cybersecurity strategies and policies. The European Commission has several initiatives in place to explore the potential of AI in the context of cybersecurity for supply chains, one of which being the FISHY project in which we participate with security sensors supporting real-time metrics for the vulnerability assessment of the IT system as part of the supply chain. Another approach in which we participate through the PIACERE project, is focusing on the security of DevOps continuous deployment both at design time and runtime.
One of the key challenges in implementing AI-powered cybersecurity solutions for supply chains is the need for strong data governance and privacy protections. As AI-powered cybersecurity solutions rely on vast amounts of data, it is essential for businesses to ensure that this data is properly collected, stored, and protected. This includes implementing strong data governance policies, such as data classification, data retention, and data access controls, as well as complying with data privacy regulations, such as the General Data Protection Regulation (GDPR) and the California Consumer Privacy Act (CCPA). Another challenge in implementing AI-powered cybersecurity solutions for supply chains is the need for skilled cybersecurity professionals. While AI-powered cybersecurity solutions can automate many cybersecurity tasks, such as threat detection and response, they still require skilled professionals to manage and oversee these solutions. This includes developing cybersecurity strategies, implementing cybersecurity policies, and monitoring network activity for signs of cyber threats.
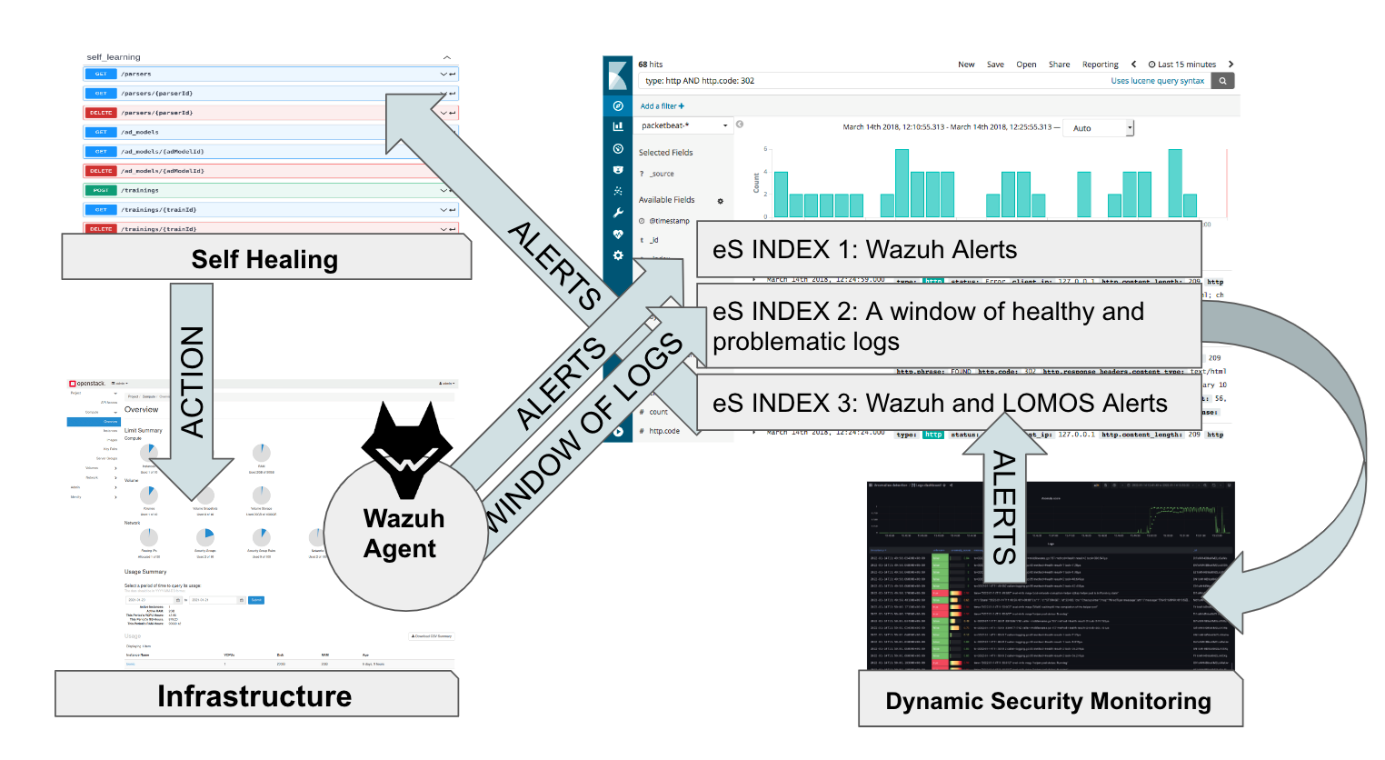
Extending the open-source security platform Wazuh, designed for real-time threat detection, analysis, and response capabilities for organizations, my colleagues at XLAB have been addressing the need for monitoring the runtime conditions of an infrastructure or an application the specific needs and requirements of DevSecOps. The team has made available a monitoring system that can detect security-related incidents and events in the deployed application's environment. This system can be deployed automatically and sends notifications to users about security alerts. Unlike other evidence-gathering tools, Wazuh agents are installed directly on the monitored infrastructure, rather than being connected to cloud interfaces. In pair with the latter static rule matching approach, the team has been developing a novel log monitoring system to complement its existing static rule matching approach. This system is designed to identify patterns and anomalies in logs without requiring manual preprocessing of raw unstructured data, identifying log templates that match logs and breaking them down into structured log templates based on a tree structure. It then observes the sequence of templates to learn what is normal behavior and provides an anomaly score for normal logs.
By combining the strengths of rule-based matching and deep learning-based anomaly detection on logs, this approach for runtime security monitoring has created a powerful monitoring system. This system can automatically detect deviations from normal operating conditions in real time, including potential security threats. The interplay between rule-based matching and deep learning-based anomaly detection allows for an effective and efficient monitoring system that combines the benefits of both approaches. During April I will have the opportunity to discuss this topic live with the audiences of ACM’s International Conference on Performance Engineering in Coimbra, Portugal, and IEEE’s International Conference on the Design of Reliable Communication Networks near Barcelona, Spain. Still in April, the PIACERE project is organizing a webinar in collaboration with the Software Forum dedicated to DevSecOps named “DevOps Innovation in Practice: New lifecycle processes, new applications” with excellent panellists such as Matija Cankar, Damian Tamburri and Andrey Sadovykh where security at runtime as well as at design time will be highlighted topics (Register Now).
You are welcome to join us to discuss the role and responsibility of AI-based systems in the resilience of supply chains. What do you think are the main concerns and challenges? Let's start the discussion here.
Attend the SWForum Webinar on DevSecOps
On April 20, the SWForum team will be offering a free webinar on "Innovation in Practice" in the context of Dev(Sec)Ops with excellent speakers that are shaping the future of the field across several projects. It will be a great opportunity to get to know more of what is going on now and to interact with the experts.
Register at https://swforum.eu/events/devops-innovation-practice-new-lifecycle-processes-new-applications